Optimizing Urban Growth: Data-Driven Best Practices for Sustainable City Planning
As cities around the world continue to experience rapid growth, sustainable urban planning has become a critical priority for local governments. With over 55% of the global population living in urban areas—a number that is expected to rise to 68% by 2050—ensuring that urban growth is well-managed and sustainable requires data-driven decision-making. Through the use of advanced technologies, data analytics, and evidence-based strategies, cities can enhance resource efficiency, improve public services, and create sustainable, resilient communities. This publication outlines how cities can leverage data to optimize urban growth and achieve sustainability, using real-world examples and case studies to demonstrate the impact of data-driven urban planning.
PUBLICATION
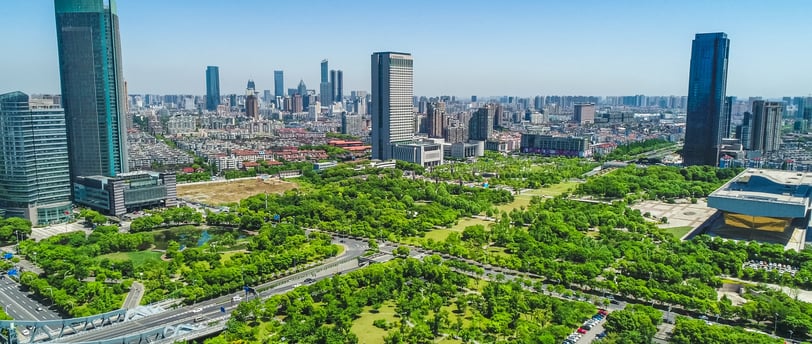
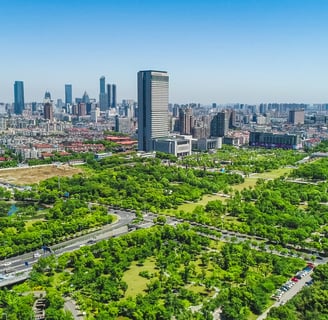
1. Integrated Land Use Planning: Using Data for Smarter Zoning
Efficient land use is a cornerstone of sustainable urban planning. With limited space and increasing populations, cities must make informed decisions on how to allocate land for residential, commercial, and green spaces. Through data analytics, municipalities can ensure that land is utilized effectively, reducing congestion and fostering diverse, mixed-use neighborhoods.
Data-Driven Insights:
GIS Mapping & Zoning Data: Geographic Information Systems (GIS) are used to analyze and visualize land use patterns, zoning regulations, and environmental factors. Cities like New York and San Francisco have used GIS tools to map zoning regulations and make data-driven decisions about where to allow new development, helping to avoid sprawl and reduce urban congestion.
Land Use Forecasting Models: By analyzing demographic data, economic trends, and infrastructure capacity, urban planners can use predictive modeling to forecast future land use needs and optimize zoning laws for long-term sustainability.
Example:
In Portland, Oregon, GIS-based zoning maps were integrated with real-time population data to develop a "20-minute neighborhood" model, which aims to ensure that residents can access basic services like healthcare, shopping, and schools within a 20-minute walk from their homes.
2. Sustainable Infrastructure: Leveraging Smart Technologies
The integration of smart technologies in urban infrastructure not only enhances efficiency but also provides valuable data that can guide decisions on energy use, waste management, and transportation.
Data-Driven Insights:
Smart Grids and Energy Management: Cities are increasingly adopting smart grids that collect real-time data on energy usage, allowing utilities to optimize energy distribution and reduce waste. For instance, Chicago uses its smart grid to monitor energy consumption patterns and make adjustments in real-time, contributing to a 15% reduction in energy costs.
Building Energy Management Systems (BEMS): These systems monitor and optimize energy consumption in buildings by analyzing data from sensors and devices. Cities like London and Paris are using data from BEMS to track energy use patterns, reduce carbon emissions, and set building standards for sustainability.
Example:
The City of Barcelona uses real-time data from smart streetlights, water meters, and air quality sensors to monitor urban systems, improving resource allocation and reducing the city’s carbon footprint.
3. Transportation and Mobility: Optimizing Transit through Data Analytics
Transportation is one of the most data-intensive aspects of urban planning. By leveraging transportation data, cities can optimize traffic flow, reduce congestion, and promote sustainable travel options.
Data-Driven Insights:
Real-Time Traffic Monitoring: Using sensors, cameras, and GPS technology, cities can collect real-time data on traffic flow and congestion. Los Angeles has deployed an extensive traffic monitoring system that uses data from over 6,000 sensors to manage traffic signals in real-time, reducing congestion and improving travel times.
Public Transit Optimization: Transit authorities can use ridership data to optimize routes and schedules. Singapore’s Land Transport Authority uses a data-driven approach to analyze commuter patterns, leading to the optimization of bus routes and the introduction of on-demand bus services in underserved areas.
Mobility-as-a-Service (MaaS): MaaS platforms aggregate data from various transportation providers to offer users tailored mobility options. Helsinki is a leader in MaaS, where users can plan and pay for multi-modal trips (bus, bike, train, etc.) in a single app, reducing car dependency and promoting sustainable transport options.
Example:
The City of London uses data from its Congestion Charge Zone to analyze traffic patterns and reduce traffic in its city center, leading to a significant decrease in air pollution and increased use of public transport.
4. Climate Resilience: Using Data to Mitigate Environmental Risks
Climate change poses a significant risk to urban areas, making it essential for cities to integrate climate resilience into their planning processes. Data-driven climate resilience frameworks allow cities to predict and mitigate environmental risks, such as flooding, heat islands, and rising sea levels.
Data-Driven Insights:
Climate Modeling and Prediction Tools: Cities are using climate models to predict future environmental changes and assess the risks of extreme weather events. New York City’s Climate Risk and Resilience Portal uses climate data to inform the city’s climate adaptation strategies, focusing on areas vulnerable to flooding, sea-level rise, and heatwaves.
Green Infrastructure Data: Data from sensors and climate models help cities determine the most effective places to implement green infrastructure, such as parks, trees, and green roofs, to mitigate urban heat islands and manage stormwater.
Example:
In Miami, the use of flood modeling tools has enabled the city to identify areas most at risk of flooding, guiding investments in flood prevention infrastructure and emergency response strategies.
5. Affordable Housing: Addressing Demand with Data-Driven Policies
With urban populations increasing, cities must address the growing demand for affordable housing. Data-driven housing strategies enable cities to better understand housing needs, market dynamics, and income disparities, facilitating the development of targeted policies that ensure affordable housing for all residents.
Data-Driven Insights:
Housing Affordability Indices: Cities like San Francisco and Vancouver use data on income levels, housing prices, and rent costs to create affordability indices, which guide policy decisions around rent control, subsidies, and affordable housing development.
Geospatial Data for Housing Development: Using GIS data, cities can identify areas with high housing demand but low supply, making it easier to target development projects that meet community needs. Seattle’s housing development plan leverages data on land availability, transit accessibility, and neighborhood demographics to prioritize affordable housing construction.
Example:
The City of Toronto uses data from the Canadian Housing Data Platform to analyze housing affordability across different neighborhoods, enabling the city to implement tailored housing policies for each area based on real-time demand and supply data.
6. Public Health and Safety: Data for Better Services
Urban health is deeply tied to the availability and quality of city services, from emergency response times to healthcare access. Data plays a vital role in ensuring that these services meet the needs of residents, especially in rapidly growing cities.
Data-Driven Insights:
Health Data Analytics: Cities like Boston are using health data analytics to monitor public health trends, predict future needs, and optimize healthcare services. For example, Boston uses data to monitor opioid-related health issues, guiding resource allocation and intervention strategies.
Crime and Safety Data: Crime mapping tools, such as those used in Chicago, leverage data from police reports, surveillance systems, and community input to allocate police resources efficiently and improve public safety.
Example:
London has implemented a city-wide data-sharing platform that integrates emergency response data, crime statistics, and hospital admissions to improve city planning and public health policy.
Conclusion: Leveraging Data for Smarter Urban Growth
Data-driven urban planning has proven to be a game-changer in managing the challenges of rapid urbanization. By leveraging data analytics, smart technologies, and predictive modeling, cities can optimize growth, enhance public services, and ensure sustainability. The insights and best practices discussed in this publication highlight how cities worldwide are using data to create more efficient, livable, and resilient urban environments.